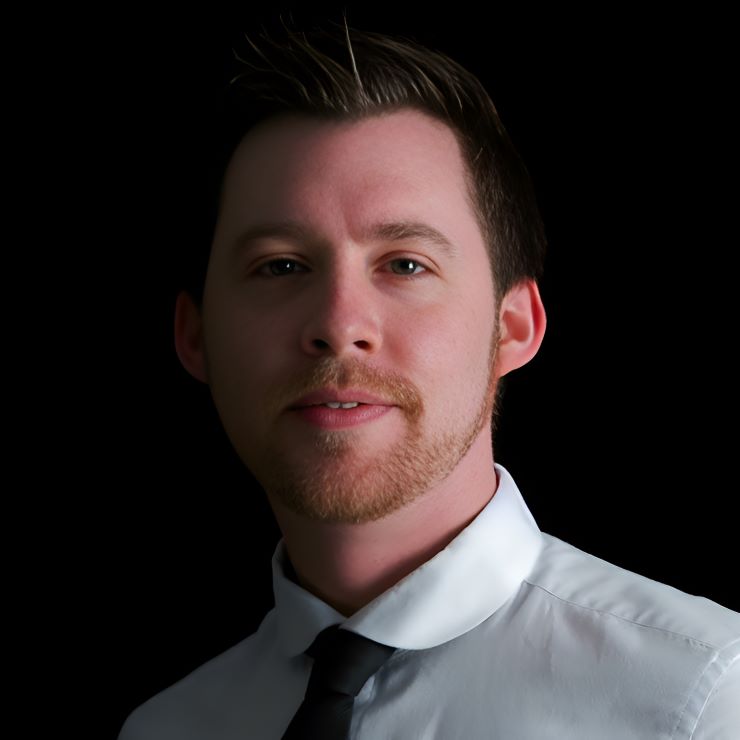
By Philip Miller, Senior Product Marketing Manager for AI, Progress
Fintech is one of those industries that is paving the way when it comes to adopting and implementing innovative technologies such as Artificial Intelligence (AI).
Heavy reliant on data, Fintech organisations are well placed to make the most out of those technologies, particularly generative AI (genAI) and machine learning which have become priorities across the sector.
The global artificial intelligence in fintech market size was valued at USD 9.45 billion in 2021 and is expected to grow at a compound annual growth rate (CAGR) of 16.5% from 2022 to 2030.
We are already seeing a wide range of use cases that are transforming the way financial organisations are building and offering their products and services, as well as how they interact with their customers.
Some of those use cases go from using genAI to improve fraud detection, risk management and investment analysis, all the way to leveraging managing learning and natural language processing for predictive analytics.
The customer service field is particularly ripe for AI disruption with tools like chatbots as well as genAI driving more ultra-personalised 24/7 services and communications to individual customers.
But the more we use AI, the more we understand its limitations and the need to proceed with caution. Using data-driven technologies without safeguards can indeed lead to significant inaccuracy and security issues.
Are AI-driven Fintechs hallucinating?
Generative AI and machine learning have become very popular among Fintech because of their ability to interpret data and enable smarter operations. This is done by leveraging large language models (LLMs) in critical applications and systems.
But these data-human interactions should be regularly monitored to reduce hallucinations and data biases and facilitate more accurate output.
Indeed, more organisations are falling victim to the famous “AI hallucinations” and what starts as a promise of a huge benefit can quickly turn into a massive failure.
Hallucinations happen when the AI generates responses that are not grounded in the data on which it was trained or on any logical basis. The information produced seems plausible but is factually incorrect and nonsensical.
These can be minor inaccuracies or completely fabricated facts, which can be really problematic as it can lead to misinformation, erroneous decisions and a lack of trust in the AI system.
RAG
Retrieval Augmented Generation (RAG) combines genAI with detailed, relevant data to deliver accurate, reliable and useful insights.
By grounding AI responses in a structured knowledge graph and validating them against a comprehensive knowledge model, RAG significantly reduces the chances of hallucinations.
RAG finds relationships and connections within the data, providing a strong framework for accurate response generation. This leads to more accurate, trustworthy and actionable insights, which are crucial for decision-making.
Particularly, semantic RAG is a technology that can help provide much needed context and insight to decrease the chances of hallucinations, improve accuracy and reduce costs. This RAG architecture basically acts as a long-term memory for the generative AI.
RAG-AI can change the game for Fintech organisations
By using RAG models, Fintech organisations can avoid falling victim of AI’s common pitfalls, and drive greater outcomes including:
● Speed of change
As the data sets collected by Fintech organisations are continuously evolving, AI models need regular updates to stay relevant and accurate. RAG frameworks are inherently flexible and model-agnostic, allowing financial services organisations to quickly adapt without significant downtime or reconfiguration.
● Improved Accuracy and Trust
RAG significantly improves the accuracy of AI-generated responses. Customers have reported accuracy rates in the high 90s—and even 100%. This accuracy builds trust in the AI system, as users can see where the AI got its answers and verify the information.
This is proving particularly important for Fintech organisations that are dealing with sensitive personal and financial information, and for which a loss in trust could prove catastrophic.
● Cost savings
RAG optimises the use of processing power, mostly because the prompts to the AI are smaller and more focused, reducing the computational load and associated costs, and making it more efficient than traditional AI methods.
Enterprise-grade RAG solutions are designed to be scalable and secure. They can handle large volumes of data and queries, while maintaining strict security protocols to protect sensitive business and customer information.
As a technology trailblazer industry, Fintech has an opportunity but also a duty to set high standards when it comes to using innovative technologies such as AI, genAI and LLMs. Investing in models and frameworks based on RAG is an easy way to lay some strong foundations for building future proof AI strategies that will result in better outcomes, while reducing potential issues such as hallucinations.