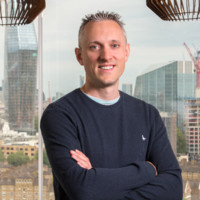
By Jamie Hutton, Chief Technology Officer of Quantexa
According to McKinsey, nearly 60% of financial services companies have embedded at least one AI capability. Among the most common are process automation, virtual agents, or text understanding. But to truly benefit from the technology, the sector needs to broaden implementations from siloed one-off products or services to be across the entire organization.
Data teams are being overwhelmed by the sheer volume of data their company is creating. The analogy we use is to imagine a river of data, and banks for the most part are siloing off portions of the river into standalone ox-bow lakes. For banks to reap the rewards of AI – estimated to have an annual potential operational RoI of 9% to 15% – they should focus on data quality, governance and usability across the whole organization. The result of this is known as Decision Intelligence; whereby decision-making is improved at every level – strategic, operational and tactical.
They’re all aware that AI is the answer to better decision making. However, as organizational leaders hurry to deploy AI and change their business model to reflect the new technology, they can’t forget the basics: Accurate and intelligent decision making comes from the best-trained AI, which starts with trusted data.
The foundation of good decisions is trusted data
AI tools are only as good as the data they’re fed. After all, the models are learning from the data itself, so if quality is low, the models will be too.
When building a predictive model, there are a few key factors that will substantially impact its accuracy.
The first is the labelling of the thing you are trying to predict – the outcome. For example, if you are trying to assess the future value or risk of a customer, you need to have trusted metrics around how value or risk is measured and have a historic view of this for your existing customers.
Secondly, it’s all about the data that is put into the model – or the “features” as a data science team would describe it. If you want to assess the risk of a customer, you need to understand as much about them as possible. Having a single view of customer is critical, even if the details they have given are inconsistent (e.g. Quantexa vs Quantexa Ltd). Then, understanding the relationships the customer has across both internal and external data gives the model a real understanding of who your customer is. This allows the model to be significantly more accurate.
Banks are aware of the need to improve decision making with the use of data. However, they often struggle to produce and maintain a contextual view of their customers. Different iterations of a name, changes in the address or multiple phone numbers can create duplications. Duplicated data dominoes into inefficiency and puts confidence in decision making at bay. It’s wasteful and expensive to have these AI and ML tools if they’re not understanding the data correctly, as business teams won’t be able to trust the outcome. Achieving decision intelligence across the business starts with data.
Data is the building-blocks of context
Research found that one in nine customer records is a duplicate of another, which is contributing to a wide mistrust in data across organizations and industries.
Banks may have data relating to the same customer but arriving from various CRM and product systems across divisions. A small business bank and a retail bank will both carry different data about one shared customer, which is not linked correctly in the system. This duplication increases substantially where an organization has grown inorganically via acquisitions. Without understanding a customer’s data across the whole enterprise, how can you make accurate decisions about that customer.
Decision makers need to be able to link together customer activity, behaviour, and relationships to fully understand whether a flag is risk. The only real way to get this full contextual picture is with entity resolution.
Entity resolution is the best infrastructure for context
Entity resolution cleans and sorts data, using AI and ML tools to interpret all the different ways one entity can be identified. Entity resolution collects records relating to each entity, compiles a set of attributes that is uniform across each entity, and from there can create a labelled link between entity and source records. This is much more effective than traditional record-to-record matching used by MDM systems, and much more efficient.
High quality entity resolution doesn’t just link your own data, but it also brings in high value external data. This can include corporate registry information – which provides real context about the performance of the business as well as the ownership structures. With models of the past, it would have been difficult to match this data accurately and reliably. An entity resolution system can link this data with ease.
Banks not yet using entity resolution are missing this contextual view of customer across both internal and external data. Entity resolution technology is vital to decision intelligence, as it removes siloed data. Instead, banks’ decision making is backed by a strong foundation of clean and contextual datasets that can then be used to train its AI models accurately and efficiently.
Regulation is catching up with the tech
This year will also be significant for AI regulation. The EU AI Act will be full introduced by 2025 as the world’s first-ever comprehensive legal framework on AI. Its intention is to encourage the use of trustworthy AI across Europe and position the EU as an innovator, while ensuring AI systems follow ethical principles.
The AI Act classifies products according to risk and adjusts scrutiny accordingly, with the intention of making the tech more “human-centric”. This is a big step in managing AI ethics, and the UK will be under pressure to follow suit to keep up with its European counterparts. The UK has already established the AI Authority that will, among many functions, ensure that relevant regulations take account of AI, ensure alignment of approach across relevant regulators in respect of AI, and undertake a gap analysis of regulatory responsibilities.
In heavily regulated industries such as banking, organizations need to ensure transparency and explainability is baked into their use of AI. Avoiding “black boxes” and having a trusted data foundation for AI will ensure you can meet current and future regulatory and compliance requirements.
The way banks deploy AI will evolve, however the best thing that any organization can do is back themselves with strong data foundation that gives a full view of their customers. With explainable and trustworthy insights, banks will be able to make strong decisions and simultaneously prepare themselves for regulatory shifts coming into play.